Cisco Duo Blog
Industry Events
Industry Events
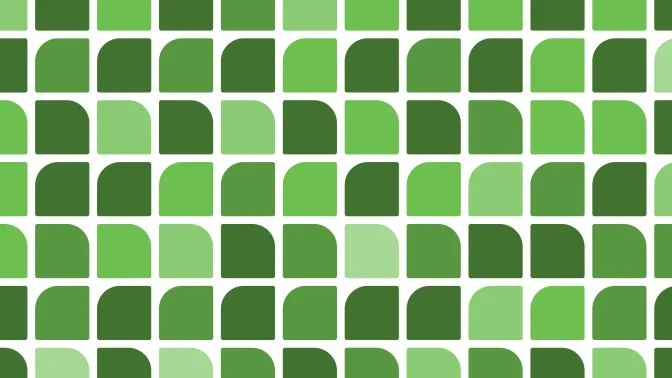
Come see Duo at Identiverse 2025
Duo dramatically expands offering with new directory and phishing-resistant functionality to address the sharp rise in identity-based attacks.
Industry Events
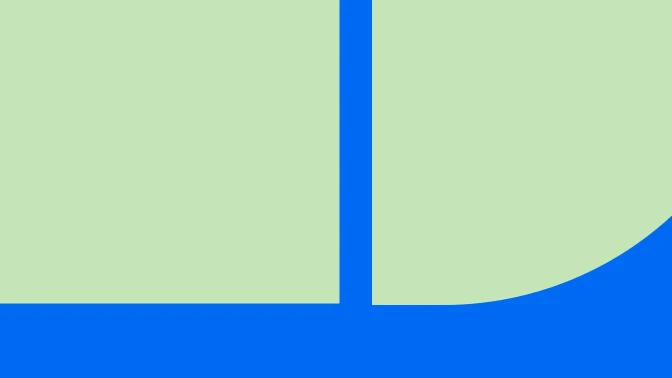
MFA adoption: The most important security metric you can measure
A multi-factor authentication (MFA) adoption dashboard provides insight into how well an organization is defended and where to secure gaps.